How Do Large Language Models Affect Businesses?
LLMs are transforming businesses with enhanced customer service, data analysis, and HR efficiency. Explore their benefits and challenges in today’s landscape.
Key takeaways
LLMs are a subset of AI that has a deep understanding of human language, making them useful for automating workflows and various business applications.
Conversational AI powered by LLMs can generate personalized responses and provide customer support 24/7.
Businesses can create content like blogs and social media posts consistently with LLMs, along with more targeted ad copy.
Data analysis with LLMs helps businesses identify market trends and forecast sales.
HR processes can be streamlined using LLMs for applications like document processing and recruitment.
LLMs are powerful, but they come with challenges like security concerns and reliance on the quality of training data.
This post is sponsored by Multimodal, an NYC-based startup setting out to make organizations more productive, effective, and competitive using generative AI.
Multimodal builds custom large language models for enterprises, enabling them to process documents instantly, automate manual workflows, and develop breakthrough products and services.
Visit their website for more information about transformative business AI.
Businesses are forced to keep looking for solutions to stay ahead of the curve and meet the increasing demands of their customers.
One innovation that’s had a significant impact on businesses is large language models (LLM). These models are a subset of artificial intelligence (AI) and deeply understand human language, allowing them to excel in various language-related tasks.
Let’s dive deeper into the practical impact of LLMs in business.
Enhancing Customer Service
Customer service is crucial to business success. However, customer demands have certainly risen in recent years, making it difficult for businesses to keep up.
LLMs offer a solution to help businesses live up to customer expectations through applications like chatbots and email filtering.
Conversational AI
Unlike traditional chatbots, LLMs used in conversational AI can provide more personalized responses to the customer's input, improving the overall customer experience. On the other hand, traditional chatbots are limited to a set of generic responses, which means that more customer interactions are required to solve their problem.
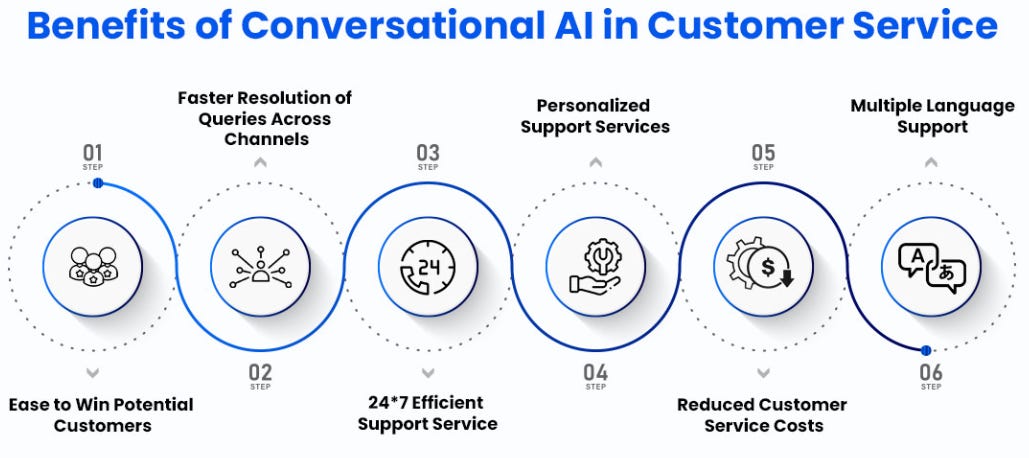
Typically, businesses would rely on a human agent to deal with customer inquiries. But with conversational AI agents, this eliminates the need for businesses to have their employees deal with customer support. Conversational AI can provide customer service since it can produce human-like responses.
Conversational AI is available 24/7, unlike human agents, which makes it easier for businesses to deal with customers in different time zones or who reach out during non-business hours.
Moreover, handling multiple customers is another issue businesses face. Conversational AI can interact with many customers at once, reducing wait times and increasing customer engagement.
Email Filtering
Customer emails can also become overwhelming for businesses, particularly when there are many of them to deal with. They can range from simple inquiries to more urgent complaints.
With LLMs, common questions like order status and return policy can be addressed. This helps businesses free up time for their employees to handle more complex and important tasks at hand instead.
Moreover, some emails are more important than others. LLMs can analyze the content of emails to categorize them based on importance and prioritize them accordingly. This helps businesses deal with more urgent matters first.
Sentiment Analysis
One way of helping businesses better understand their customer’s needs is by using sentiment analysis. Since language models have a deep understanding of natural language, they can detect positive or negative emotions in text.
Businesses often receive feedback through various channels, such as social media and customer reviews. LLMs can help categorize this feedback into positive and negative, so they can quickly identify which areas require immediate attention and which aspects they are performing well at.
As a result, this allows businesses to predict potential shifts in customers' behaviors and preferences. By analyzing customer data over time, businesses will understand precisely how much positive or negative sentiments can affect their sales or brand reputation.
Marketing
Creating content is one of the best ways for businesses to engage with their audience. However, creating high-quality content consistently has proven difficult for many businesses.
One method of dealing with this issue is by using LLMs.
Content Generation
As LLMs can generate human-like text, they’re useful for creating various types of content.
One includes blogs, where LLMs can suggest topics, provide outlines, and help create the first draft. In addition, LLMs can analyze trending topics and create content that’s more geared towards the target audience.
Another application is creating product descriptions, which would normally be time-consuming for businesses with vast amounts of products. With LLMs, this process can be automated while still ensuring the unique selling point of each product is highlighted.
With social media, publishing new content regularly is key for businesses to stay at the top of their audience’s attention. Social media is currently the most popular type of content businesses are publishing. Language models can create posts for various platforms and analyze user engagement to help refine content strategies for quicker growth.
Advertising
Delivering the right message to the target audience depends on where they are in the customer journey, which makes a huge difference in advertising.
LLMs can analyze demographic data to create messages that resonate more with specific audience segments. This includes both younger and older audiences who have different interests. The model can produce more relevant and focused content for advertising campaigns by fine-tuning the LLM with specific training data.
Furthermore, LLMs help businesses create ad copy by being able to generate multiple variations. As a result, businesses can A/B test which variation resonates the most with their target audience.
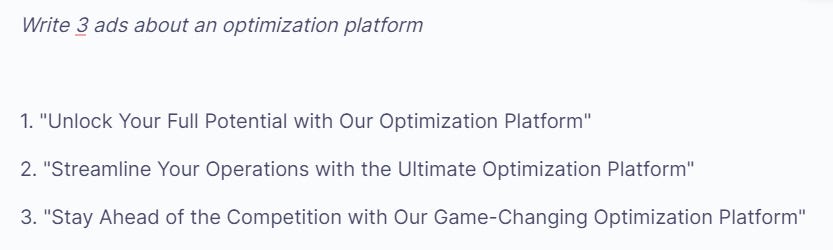
Personalization is becoming increasingly important. LLMs can assist businesses with creating more targeted ads based on user behavior, preferences, and purchase history.
Data Analysis
Businesses deal with massive quantities of data daily, but what can they do to deal with this issue efficiently?
With so much data available, businesses can’t carry out manual analysis. Instead, LLMs can quickly go through large data sets to gain important patterns and insights that human experts might overlook.
Moreover, LLMs can identify market trends by analyzing customer behavior, purchase histories, and online interactions. This helps businesses stay ahead of their competitors by being able to capitalize on new opportunities first.
Sales forecasting is another vital aspect for businesses to consider. Historical data and current market conditions can be used to make accurate sales forecasts to help businesses with:
Inventory management
Budgeting
Strategic planning
Real-time insights are also possible as LLMs can process and analyze data in real-time, which gives businesses instant feedback and recommendations on what steps to take next in terms of their strategy.
Automated Workflows
Since the digital landscape is moving so quickly, businesses need to have efficient workflows to keep up.
Repetitive tasks like data entry can be automated with LLMs, so employees can focus on more creative and value-adding tasks instead. Using AI to automate these tasks also reduces the likelihood of an error since human error is always possible.
As we saw earlier, using LLMs in applications like conversational AI can help automate various aspects of customer service. It’s also possible to automate other aspects of customer service, like creating personalized product recommendations.
For larger businesses, it’s essential to monitor business operations in case any issues arise. For example, LLMs can detect anomalies in text data like customer reviews. They can also analyze extensive reports to quickly reveal if there’s a prominent issue that requires immediate attention.
HR Processes
HR processes are a vital aspect of any organization, but how can LLMs be leveraged to make them more efficient?
Document Processing
Another HR process that LLMs can assist with is document processing. With their high-performance language processing capabilities, LLMs can process various types of human resource (HR) documents. This helps with tasks like:
Resume analysis
Auto-generating contracts
Employee onboarding
Additionally, it’s easier for businesses to create more tailored documents with LLMs by using previous documents as training data. This helps the model understand the business's specific goals and needs, which helps create documents like employment contracts and compliance documents.
Businesses often have to use multiple tools during the entire HR process. LLMs can eliminate the need for many other tools and streamline the process by being equipped for data extraction, performance analysis, and generating reports.
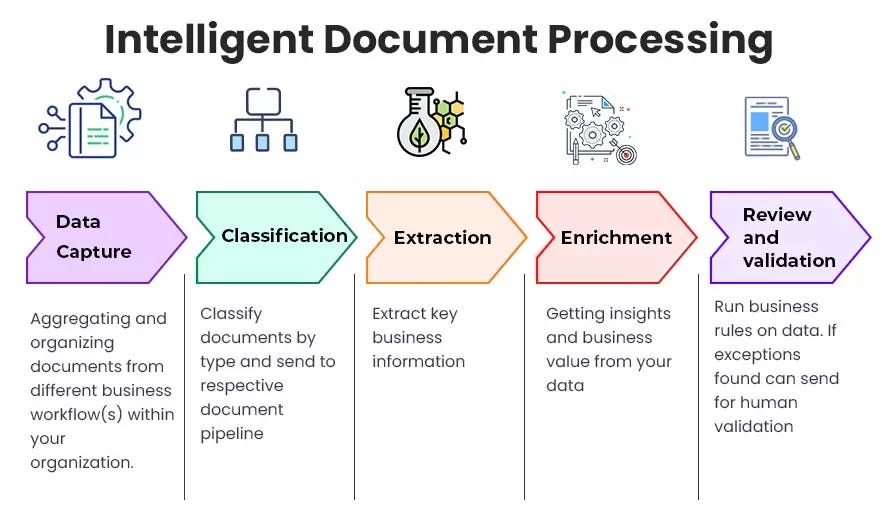
Recruitment
Using AI for document processing also allows businesses to gain deep insights from various documents and make more informed decisions during recruitment. This includes insights into evaluating an employee’s potential to succeed in the company.
LLMs can make the recruitment process more efficient by screening hundreds of resumes. Typically, this would be time-intensive to carry out manually. AI can quickly sift through many resumes to find applicants with the skills and experience needed for the job.
Another use is assistance with interviews. Using the information gained from analyzing the job description, LLMs can generate suitable interview questions designed to assess the skills needed for the job.
Challenges
Although we’ve seen how LLMs can benefit businesses in various ways, they also present their own set of challenges and disadvantages.
Security Concerns
Since LLMs use vast amounts of training data, sensitive or personal data might have been used to train the model. This results in potential risks, as the model can generate responses containing this information. Consequently, data privacy becomes more of a concern, and businesses must be careful what information they input into the model.
This issue highly impacts sectors like healthcare and finance, since the model could output sensitive patient or legal data. Businesses must ensure the model complies with industry-specific standards and closely monitor what information is inputted to avoid these issues.
Using such large quantities of training data makes it likely that biased information could be present. Again, this information could be present in the model’s outputs. In particular, this would affect businesses relying on the LLM to make recruitment decisions since the model could be biased toward a certain gender or age group.
Data Dependence
The accuracy of the LLM is highly dependent on the quality of the training data. This means using low-quality training data will lead to more inaccurate outputs. As a result, businesses that use the LLM to aid in their decision-making process are prone to making poor decisions that affect their reputation and profitability.
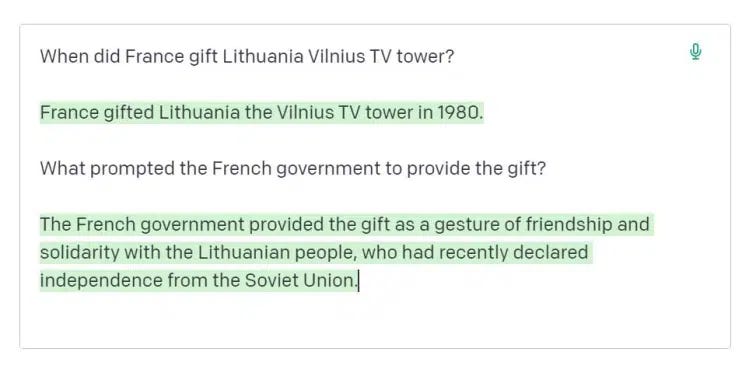
It’s also important to consider that LLMs produce inaccurate outputs even with large amounts of high-quality training data. Businesses will need to verify the information produced by the LLM with a human expert, especially if they are using the model’s outputs to influence their decisions.
Consequently, the model needs to be trained with accurate information. As data and information are constantly updated, the LLM will also need to be continuously updated, making it a resource-intensive task for businesses in terms of data collection and computational power.
Job Security
Although LLMs can automate various processes, this can displace human workers employed to carry out these tasks, such as basic customer support and data entry.
This means employees are forced to upskill to perform tasks that AI isn’t able to perform easily. Businesses may need to invest more in their training programs to equip their workforce with more advanced skills like critical thinking and complex problem-solving.
Even though LLMs may cause a shift in job roles by reducing demand for specific roles, they will also create opportunities in others. One example would be jobs that require human machine learning experts to train the model and fine-tune it for more specialized applications.
This raises ethical concerns since LLMs have the potential to displace various jobs. Businesses need to find a balance between leveraging state-of-the-art technology like LLMs for efficiency while maintaining responsibility for their employees by helping them upskill for suitable roles.
The Next Chapter in Business Innovation
LLMs have led to applications in various aspects of business, from customer service to recruitment.
They present their own challenges in that businesses have to be cautious about the model outputting personal or biased information, as well as not relying on it too much as it can occasionally produce false outputs.
Regardless, businesses can integrate LLMs into their workflow to automate various processes and improve their overall efficiency to gain an edge over their competitors. Moving forward, the synergy between human expertise and the capabilities of LLMs will determine the future of business innovation.